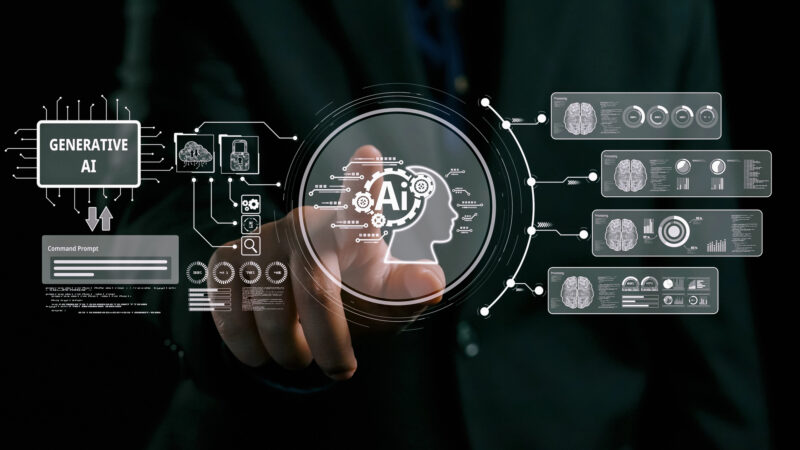
Understanding different AI systems and AI models is essential to unlock the true potential of the thousands and thousands of varying AI tools. In addition, two AI technologies revolutionizing how organizations across all industries operate are large language models (LLMs) and generative artificial intelligence (AI).
While LLMs and generative AI tools have inherent overlaps, there are key differences in how these technologies function, how they benefit end users, and how they can unintentionally cause challenges along the way.
An expert in AI systems is your best resource for understanding these complex artificial intelligence technologies and their uses.
C1M is at the forefront of evolving AI models and AI systems. We can help you better understand artificial intelligence and, more importantly, guide real-life AI system implementation that will demonstrably improve your organization’s efficiency and revenue.
However, it helps to have a baseline understanding of what LLM and generative AI systems entail and how they work. So, start your LLMs and generative AI research with the following overview of these two AI models’ backbone functionalities, noticeable benefits, and potential limitations.
Table of Contents
How Does Generative AI Work Behind the Scenes?
Examples of Generative AI Models
Real-life Applications of Generative AI in Various Fields
What are Large Language Models (LLMs)?
The Key Differences Between LLMs and Generative AI
Ethical Considerations for Using Generative AI and LLMs
Generative AI and Large Language Models with C1M
What is Generative AI?
Generative AI is broadly defined as artificial intelligence that is designed for original content generation, and this content creation can take many forms.
When most end users think of generative AI-powered content creation, they naturally envision the ability to produce coherent blogs and articles and handle other straightforward text- generation tasks.
However, generative AI refers to a much deeper level of content creation that goes far beyond text generation alone.
The generative AI model can effectively generate content in text, image, music, code, and even video form, by consuming vast amounts of data and then employing complex machine-learning algorithms to understand patterns and formulate an output.
How does generative AI work behind the scenes?
Because generative AI has such a broad scope when it comes to types of content generation, it’s fueled in a wide array of ways, depending on the content summarization required.
These methods or techniques include recurrent neural networks (RNNs), generative adversarial networks (GANs), and/or transformer architecture, which is the “T” that you’ll find in the familiar ChatGPT application.
However, all of these techniques, as well as the extensive datasets and input data utilized, are dependent on the end goal of the content generation. For example, a generative AI model designed for image generation will tap into millions of image-based datasets found in all corners of the internet for eventual image synthesis or image output. A generative AI model designed for music composition will perform similar research and dataset scouring but with audio files and videos.
Simply put, the reach of generative AI is deep, and the best generative AI model or generative AI applications to lean on will depend on your content generation ambitions.
Examples of generative AI models
Did you know there are thousands of generative AI models for content generation? Well, there really are.
With that being said, some AI tools that rely on generative AI are more common and familiar than others, and examples of these widely used generative AI tools include the following.
DALL-E
Developed by OpenAI, this generative AI platform can create detailed and unique images based on textual data. Additionally, it is powered by understanding contextually relevant text and the relationships between words.
Dream Studio
This is a generative AI platform that is popular for music composition. It utilizes and employs machine-learning algorithms to analyze patterns in music data and then creates original compositions that are based on a user’s input and varying style preferences.
Runway
As another generative AI platform that goes miles beyond text-focused tasks, this generative AI tool is popular with professionals in a range of creative fields. It can create realistic images or photo manipulations, automate videos and filmmaking ventures, and more.
Real-life applications of generative AI in various fields
Generative AI has an ever-evolving range of uses, and it’s often found in creative fields as a supplemental tool for content generation in all its forms.
However, outside of the creative realm, generative AI can have applications in virtually every common and customer-oriented industry. For example, in financial fields, generative AI can analyze vast amounts of market trends to provide individualized or far-reaching financial advice. It can automate document review and analysis in business and legal fields, deciphering tons of textual data or images like graphs into easy-to-digest insights.
In marketing, generative AI is a game changer for content creation in all its forms. Since generative AI encompasses text data as well as images, videos, and even music, marketing professionals have a range of tools at their disposal for generating multi-media and resonating marketing campaigns – from logos and brand identity essentials to newsletters to TikTok videos.
What are large language models (LLMs)?
Large language models (LLMs) are a type of generative AI that focuses specifically on language-related and text- based tasks. Unlike the broader concept of generative AI, which has a deep understanding of content and content generation in virtually all forms, a large language model is powered by text data and textual analysis to create text generation that mimics human language.
LLMs are a special type of AI model that relies on natural language processing (NLP) to understand and create humanlike text. This humanlike text can be used in a multiple of ways outside of the confines of straightforward text generation – like constructing an essay or article – and text-based tasks can be implemented in the customer service, marketing, and communications arenas.
Simply put, both generative AI and LLMs use vast amounts of data for content generation. However, large language models can tackle a myriad of language related tasks that span from human-like text generation to speech recognition.
How do large language models work behind the scenes?
All AI models rely on sophisticated algorithms to work. On the other hand, since large language models are designed for handling language related tasks, they use deep learning to understand and interpret human language.
Behind the scenes, large language models tap into diverse training data, such as books, research papers, Wikipedia entries, and other text data that is abundant on the Internet.
Large language models are built on a kind of neural network called a transformer model, and transformers process entire sequences of this text data in parallel, which leads to faster training.
An encoder and decoder in the transformer model extract meaning from this text data to better understand the relationships between words and phrases. After all this text data training, large language models are continually fine-tuned so they can become better at generating coherent content and responses and making accurate predictions as needed – such as answering questions in a chat.
Familiar examples of large language models
There are many large language models, and that number is growing rapidly. A sample of wholly recognizable and familiar large language models – which you may have even used at some point today – includes the following.
- GPT-3 and GPT 4 – Developed by OpenAI, the GPT-3 and GPT 4 (with GPT standing for Generative Pre-trained Transformer) are two of the most prominent and widely used large language models. These large, successive language models produce coherent, contextually relevant text in various applications, including chatbots, content summarization, content generation, and language translation.
- BERT (Bidirectional Encoder Representations from Transformers) – Developed by Google, BERT is another large language model with 340 million parameters. BERT is a pre-trained model focusing on understanding language and processing natural language data. As such, it can be used in many different applications like text data classification, language understanding, and question-answering AI systems.
- LLaMA (Large Language Model Meta AI) – Trained in 20 languages, this large language model by Meta has the capability to have conversations and engage in creative writing, making it one of the more versatile language models.
- PaLM 2 – Powering the Google Bard chatbot, this large language model focuses on language understanding, language translation, and language generation, which leads to enhanced performance in tasks such as text completion, natural language understanding and modeling, and document classification.
Real-life applications of Large Language Models (LLMs) in various fields
You’ll find multiple uses for Large Language Models (LLMs) in industries that benefit from these types of technology tools. Most notably, LLMs are helpful for streamlining customer communications and customer service operations, thanks to their language understanding and ability to detect language patterns in all forms. As such, common LLMs like chatbots can create human-like text and contextually relevant text, making it easy to field both simple and more in-depth queries from customers.
LLMs can also be helpful for language translation applications, allowing organizations to reach new global markets that require language translation to understand text data.
LLMs can also be used for a range of other text-based tasks, specifically text generation for website content, articles, research papers, marketing emails and initiatives, and more.
The key differences between LLMs and Generative AI
The key differences between LLMs and generative AI boil down to their capabilities.
Because large language models are a subset of generative AI, these AI models are focused specifically on text generation. Generative AI, however, has a broader range capable of widespread content generation, including images, audio, video, and even code generation. As such, a generative AI model uses many more data sources besides straightforward text data.
Both generative AI and LLMs are artificial technologies that utilize machine learning, natural language processing, and extensive text data (and other data) to generate content. Generative AI has less specialized expertise and larger scope, while LLMs focus on language translation and text generation.
Ethical Considerations for Using Generative AI and LLMs
Since artificial intelligence is a new and evolving technology, there are ethical concerns and other challenges to consider when implementing generative AI or large language model applications. These include the following:
Deepfakes and content authenticity (aka, that’s not Morgan Freeman)
Because generative AI is becoming so powerful, the AI landscape is creating fake images, videos, and content that leads to misinformation throughout the internet. An infamous and recent example is a 2024 AI-generated video of Morgan Freeman that went viral, sparking concern in all corners of the globe about how chillingly realistic AI-generated content can be.
Plagiarism
Given that end users do not automatically have access to sophisticated algorithms and training data that powers generative AI, plagiarism is an ever-growing concern. As such, it’s essential to review all text generation to avoid reputational and possible financial damage to your organization.
Inaccuracy
Again, the effectiveness of a generative AI system is dependent on training data and data availability. Therefore, as everyone knows, not all data on the internet and in the AI landscape is accurate. This is precisely where human intervention is required because artificial intelligence doesn’t care about the quality, accuracy, or moral concerns about the ensuing content or text created – artificial intelligence just cares about the content generation itself.
Potential job displacement… although not really
One of the biggest misconceptions when it comes to artificial intelligence is that it will replace jobs across multiple industries. However, that is not the case. Instead, generative AI and large language models (LLMs) are to improve communications and efficiency and not replace your current operations with a whole new system that relies on generative AI models alone.
As stated, artificial intelligence doesn’t have the social, emotional, or moral know-how to differentiate between quality text and content or problematic content. Therefore, generative AI can still handle only relatively limited task complexity. In other words, generative AI and LLMs are a tool for humans, but not a replacement for humans by any means.
Generative AI and large language models (LLMs) with C1M
We’re at the forefront of artificial intelligence and can help you implement AI tools and systems into your operations to enhance your team’s productivity and bottom line.
Reach out to us today to start the conversation. Learn about the many generative AI and LLM resources at your disposal. From better communications and customer service to more effective (but less expensive) marketing strategies, C1M is the leader in using the latest artificial intelligence advancements and technology to your best benefit.
Ready to implement generative AI and LLMs for your business? Let’s transform your operations with advanced technology.